Discrete Applied Mathematics Seminar by Hongwei Jin:Beyond Graph Learning: Advances in Robust Optimization and Topological Deep Learning for Complex Data Structures
Speaker: Hongwei Jin, postdoctoral appointee,Argonne National Laboratory
Title: Beyond Graph Learning: Advances in Robust Optimization and Topological Deep Learning for Complex Data Structures
Abstract: Recent advancements in graph-based machine learning have expanded beyond traditional graph neural networks (GNNs) to address key challenges in robustness, higher-order relationships, and diverse applications. This work synthesizes three emerging directions in the field: robust graph learning, topological deep learning, and real-world applications. We first address the vulnerability of GNNs to adversarial attacks through a novel certificate of robustness framework, incorporating Lagrangian dualization and permutation-invariant constraints via Orthogonal Gromov-Wasserstein distance. Second, we explore the extension from pairwise interactions to higher-order relationships through Topological Deep Learning (TDL), enabling the processing of complex n-body relations in various domains. Finally, we demonstrate the practical impact of these theoretical advances through applications in power grid systems and computational workflow anomaly detection. This work bridges the gap between theoretical robustness guarantees and practical applications while extending the capabilities of graph-based learning to more complex topological structures.
Discrete Applied Math Seminar
Event Contact
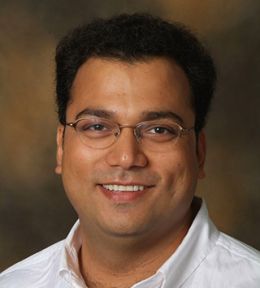