Computer Science Researchers Secure Contract to Develop Authentic Authorship Attribution and Anonymization Methods
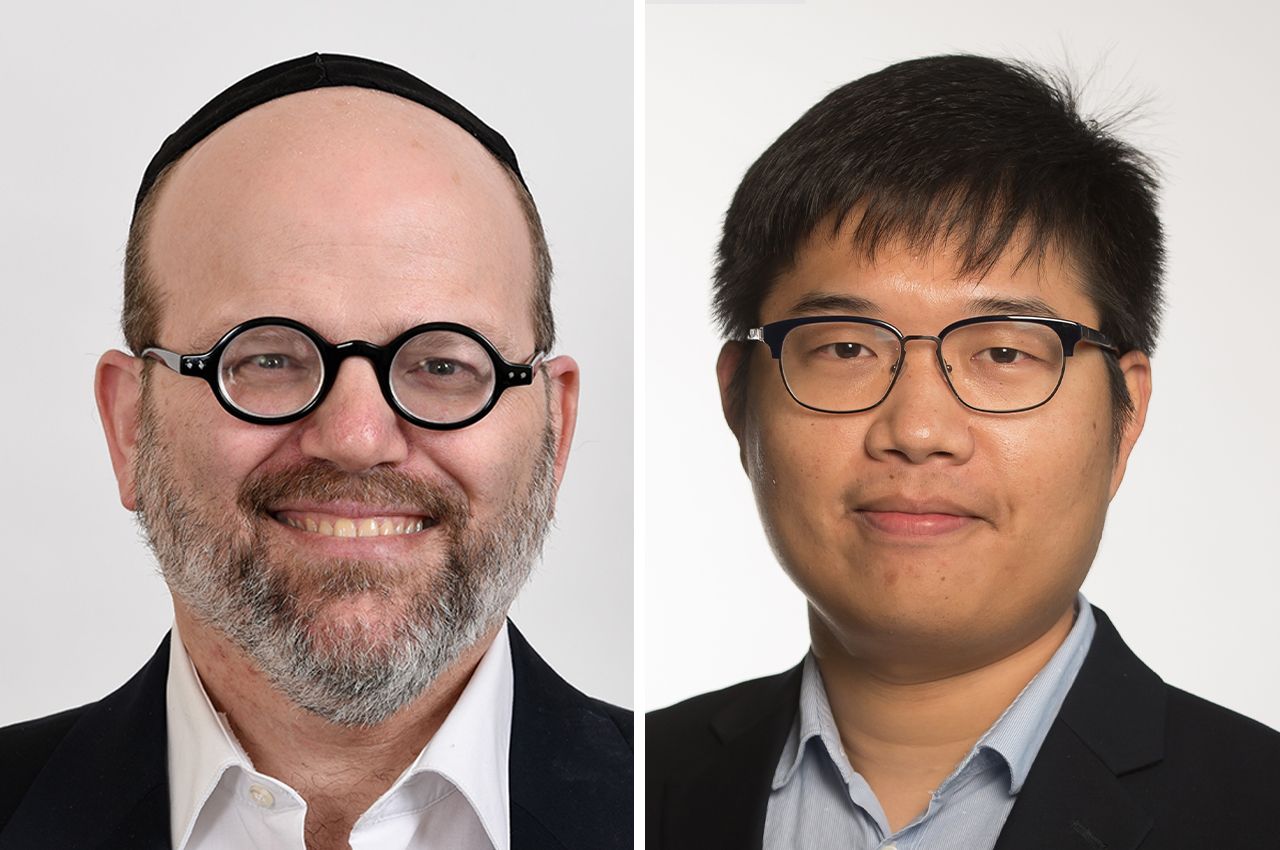
Researchers at Illinois Institute of Technology have combined efforts with researchers at Charles River Analytics, Rensselaer Polytechnic Institute, Aston University, and the Howard Brain Sciences Foundation to secure a contact for a project that combines natural language processing and machine learning to develop a versatile, theory-based approach to authorship attribution and obfuscation.
Shlomo Argamon, professor of computer science and chair of the Department of Computer Science at Illinois Tech, and Kai Shu, Gladwin Development Chair Assistant Professor of Computer Science at Illinois Tech, received $1.6 million of an $11.3 million contract from the Human Interpretable Attribution of Text Using Underlying Structure program of the Intelligence Advanced Research Projects Activity to develop AUTHOR: Attribution, and Undermining the Attribution, of Text While Providing Human-Oriented Rationales.
AUTHOR promises to capture the writing style of specific uncredited authors through natural language processing and machine learning techniques to create stylistic fingerprints. On the flip side, AUTHOR will be used to develop authorship privacy, anonymizing identities of authors, especially when their lives are in danger.
“There are a number of different types of authorship attribution tasks,” Argamon says. “One is where there is a particular author who we want to identify in different texts. Another is where we have a specific text which we want to attribute to one of a number of candidate authors. A third is simply to determine when two texts have been written by the same person or not.”
Authorship analysis has always played an important role in intelligence and law enforcement. The urgency has been amplified by malicious actors using the virtual megaphone of the internet to exploit anonymity, and growth of machine-generated disinformation on social media. Identifying machine-generated text, or better yet specific machines, is another challenge.
“With large language models, such as GPT-3, it is possible that human-like texts can be generated from these ‘bots,’” Shu says. “Our work will explore deep generative models and style transfer techniques to explore the boundary of human-written and machine-generated texts.”
Current methods of authorship analysis and obfuscation (anonymization) have limitations. A major problem in regards to attribution is identifying authorship when the questioned document is of a different type than the known documents. Each person’s characteristic style of writing comes out in different ways depending on the document that they write such as a personal letter, a persuasive essay, an academic article, a blog post, or a short story. Each of these document types has a style that modifies the author’s own style.
“The best current methods do very poorly when test documents are of a different type than the training documents,” Argamon says. “We will develop author models that incorporate such stylistic domain dependence to enable more generally effective attribution.”
Obfuscating authorship involves changing the way a text is written while maintaining its meaning. Recent advances in machine learning have made it possible for computers to generate text that is fluent in a specific language, but these methods do not have any real understanding of the meaning of the text. This often leads to generation of false or meaningless statements.
“Our work will explore integrating deep learning with semantic knowledge representation to create useful representations of both the style and content of a text,” Argamon says. “That way the style can be changed while keeping the content constant.”
AUTHOR is expected to develop identifying fingerprints by evaluating grammar and singling out discourse features, such as how arguments are constructed, combined with traditional methods such as word use and frequency.
Unlike other algorithms, AUTHOR also will explain how author identification has been established.
The project has a wide range of promising applications including identifying counterintelligence risks, combating misinformation online, fighting human trafficking, and even figuring out authorship of ancient religious texts.
Although computational authorship analysis has gained recognition within the last decade, Argamon has been conducting research in the field for more than 25 years.
“IARPA’s HIATUS program, which funds AUTHOR, is the first research funding program that has focused on this problem, and it promises, through our work and that of the other teams, to produce fundamental advances in our understanding of authorship as it is represented in texts,” he says.