New Research Aims to Highlight and Track Uncertainty in Data
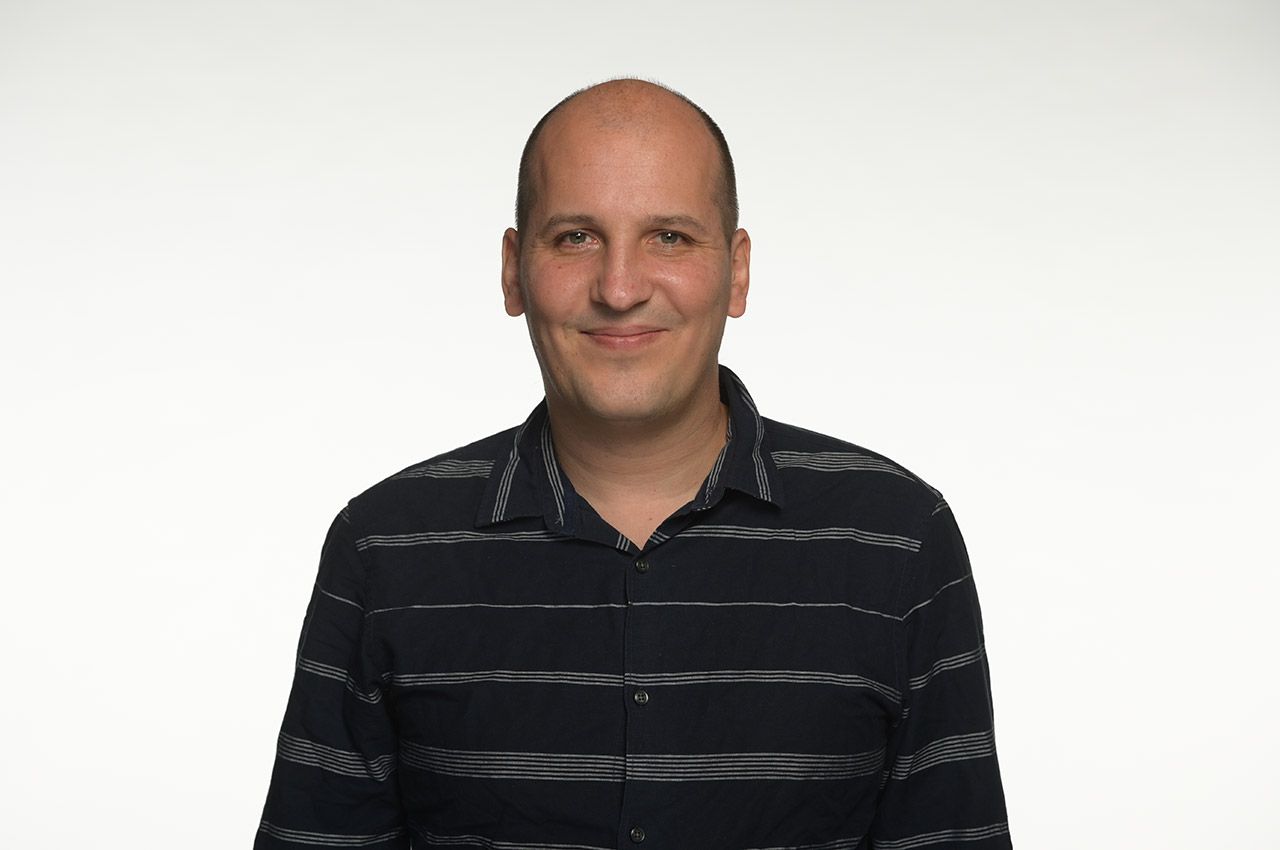
Uncertainty is a prevalent hazard for data analysts. Data uncertainty takes many forms, including missing values, sensor errors, biases, outliers, outdated information, and many others. If ignored, uncertainty can lead to incorrect analysis results, which in turn can have severe real-world implications such as wrongful convictions, denial of loan applications, and misdiagnosis of medical conditions. However, Boris Glavic, associate professor of computer science at Illinois Institute of Technology, is developing new techniques to identify and track uncertainty in data.
With University at Buffalo Department of Computer Science and Engineering collaborators Oliver Kennedy, associate professor, and Atri Rudra, professor, the team’s research focuses on identifying uncertainty in big data sets and analyzing how uncertainty affects analysis results.
“The main reason why tracking uncertainty isn’t feasible now is computational complexity,” Glavic says. “The challenge is to develop a technique that is sufficiently efficient without forsaking guarantees.”
Classical deterministic data management does not track uncertainty and, thus, data analysts have no means to judge how it affects the quality of results. Probabilistic and incomplete databases have been developed to manage uncertainty; however, these techniques are too slow for real-world applications.
“The challenge is to balance between efficiency and precision,” Glavic says. “While great progress has been made in this field, the high computational complexity of these approaches prevents their real-world adoption.”
The foundation of the research is a novel data model called uncertainty-annotated databases that enriches data with uncertainty labels. This model, that is developed by Glavic and his collaborators, will combine the efficiency and ease-of-use of deterministic data management with precise notes to alert the analyst of uncertainty.
“The main innovation is to efficiently track how uncertainty propagates through analysis and data curation processes,” Glavic explains. “At some point uncertainty needs to be modeled or identified and mapped into our uncertain data model. To simplify this, we have developed a general purpose strategy for exposing uncertainty that stems from choices made in data cleaning and curation.”
With funding from a National Science Foundation grant, the team aims to develop new software that will expose uncertainty during data analysis and curation. The software will not only identify and track uncertainty, but also determine what caused the uncertainty.
“I am very excited about two aspects,” Glavic says. “There are some really interesting and challenging technical and theoretical problems that need to be solved, and this work has the potential to address many of the real world problems caused by uncertainty in data.”
Disclaimer: The opinions, findings, and conclusions or recommendations expressed are those of the researcher(s) and do not necessarily reflect the views of the National Science Foundation.
Photo: Associate Professor of Computer Science Boris Glavic