Remembering Karl Menger
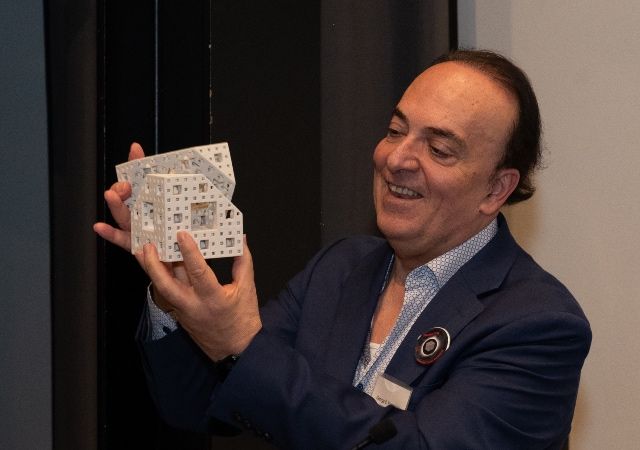
Menger Lecture 2024
“From Physics-Informed Machine Learning to Physics-Informed Machine Intelligence: Quo Vadimus?”
by George Karniadakis, Charles Pitts Robinson and John Palmer Barstow Professor of Applied Mathematics at Brown University
6 p.m. on Monday, April 8, 2024
We will review physics-informed neural networks (PINNs) and summarize available extensions for applications in computational science and engineering. We will also introduce new neural networks that learn functionals and nonlinear operators from functions and corresponding responses for system identification. Finally, we will present first results on the next generation of these architectures to biologically plausible designs based on spiking neural networks that are more efficient and closer to human intelligence. We will present applications of physics-informed machine learning in engineering, physics, and biomedicine.
Watch the 2024 Menger Lecture
Made possible with the generous support of the Menger family; Department of Applied Mathematics, Illinois Institute of Technology; and the Menger Fund.
The Department of Applied Mathematics at Illinois Institute of Technology celebrates the life, work, and legacy of Karl Menger with the annual Karl Menger Day. The day’s events features the Menger Lecture, which a prominent researcher in the field of applied mathematics is invited to speak about their recent work. The guest lecturer typically holds a related seminar, and meets with current students.
The day’s events also highlights the research conducted by current students enrolled in the department's academic programs. Students are invited to present their work to Menger Day visitors through a poster session. The Menger Awards presentation recognizes the exceptional scholarship by current students.
Karl Menger was a faculty member in the Department of Mathematics at Illinois Tech from 1946 to 1971, who influenced many students, fellow faculty members, and friends during his lifetime. Regarded as one of the finest mathematicians of the twentieth century, he made significant contributions to the fields of dimension theory, probability, economics, ethics, geometry, and calculus.
From Physics-Informed Machine Learning to Physics-Informed Machine Intelligence: Quo Vadimus?
Abstract: Physics-informed neural networks (PINNs) and neural operators like DeepOnet have formed
the foundations of Scientific Machine Learning (SciML) with applications across all domains in science,
engineering and biomedicine. Here, will present a mathematical view of these neural networks, analyzing
their convergence, and provide interpretations for their successes and failures in some prototype
benchmark problems but also realistic applications. Specifically, we will discuss how PINNs can be used
to tackle the curse-of-dimensionality, and how DeepOnet can be used to obtain in real-time 3D flow fields
for a large distribution of parameters. We will also discuss the limitations of these methods for predicting
long-term dynamics even for simple problems for which conventional methods succeed.
About the Lecturer
George Karniadakis’s research interests include diverse topics in computational science both on algorithms and applications. A main current thrust is physics-informed neural networks (PINNs) for stochastic multiscale modeling of physical and biological systems. Particular aspects include stochastic differential equations, modeling uncertainty with polynomial chaos, multiscale modeling of biological systems, atomistic/mesoscopic modelling – dissipative particle dynamics, low dimensional modeling - gappy data - data assimilation, spectral/hp element and discontinuous Galerkin methods, turbulent drag reduction, DNS/LES of turbulence in complex geometries, flow-structure interactions, micro-transport and dynamic self-assembly, flow and heat control applications, parallel computing, and interactive/virtual reality computer graphics.
George Karniadakis received his S.M. (1984) and Ph.D. (1987) from Massachusetts Institute of Technology. He was appointed lecturer in the Department of Mechanical Engineering at MIT in 1987 and subsequently he joined the Center for Turbulence Research at Stanford / Nasa Ames. He joined Princeton University as an assistant professor in the Department of Mechanical and Aerospace Engineering and as associate faculty in the Program of Applied and Computational Mathematics. He was a visiting professor at CalTech (1993) in the Aeronautics Department. He joined Brown University as associate professor of applied mathematics in the Center for Fluid Mechanics on January 1, 1994. He became a full professor on July 1, 1996. He has been a visiting professor and senior lecturer of ocean/mechanical engineering at MIT since September 1, 2000. He was visiting professor at Peking University (Fall 2007 & 2013). He is a fellow of AAAS (2019), the Society for Industrial and Applied Mathematics (SIAM, 2010-), fellow of the American Physical Society (APS, 2004-), fellow of the American Society of Mechanical Engineers (ASME, 2003-) and associate fellow of the American Institute of Aeronautics and Astronautics (AIAA, 2006-). He received the SIAM/ACM Prize in Computational Science & Engineering (2021), the SIAM Ralf Kleinman Award (2015), CFD award (2007) and the inaugural J Tinsley Oden Medal (2013) by the US Association in Computational Mechanics.